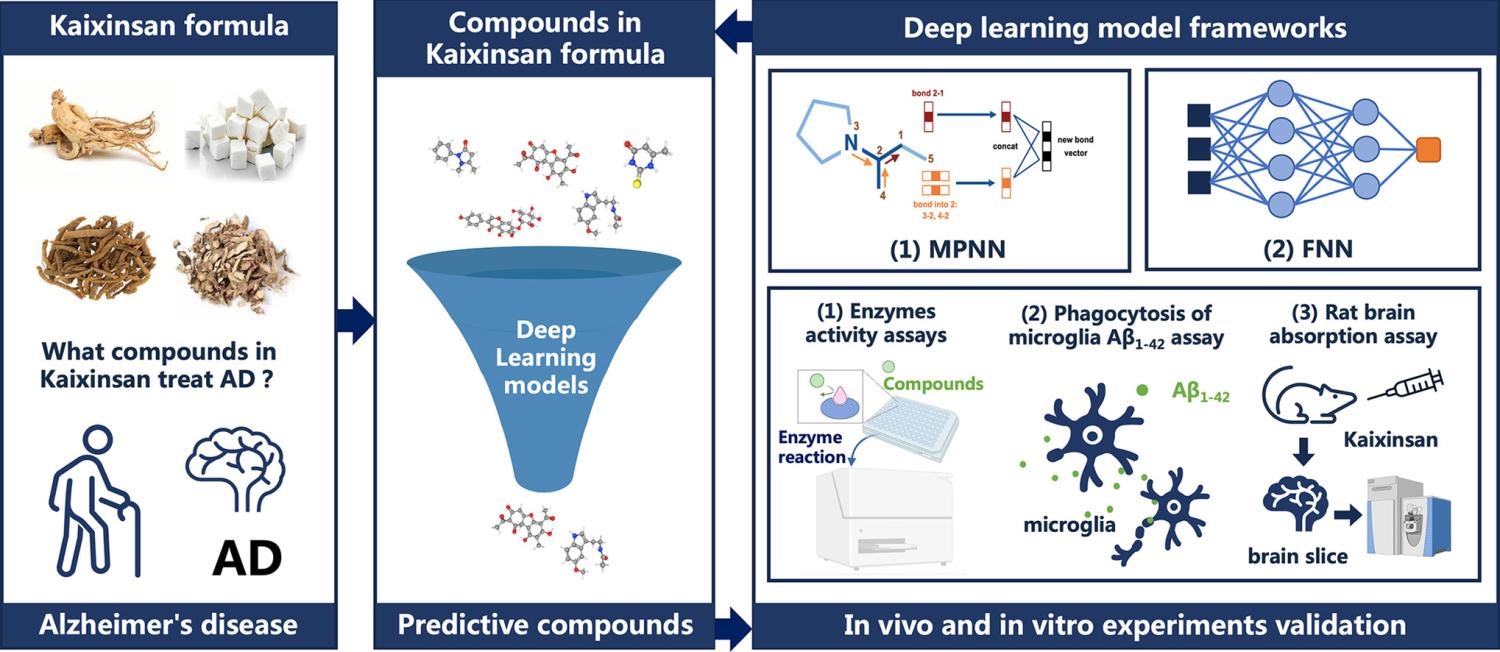
Alzheimer’s disease (AD) is gradually increasing in prevalence and the complexity of its pathogenesis has led to a lengthy process of developing therapeutic drugs with limited success. Faced with this challenge, we proposed using a state-of-the-art drug screening algorithm to identify potential therapeutic compounds for AD from traditional Chinese medicine formulas with strong empirical support. We developed four deep neural network models for AD drugs screening at the disease and target levels. The AD model was trained with compounds labeled for AD activity to predict active compounds at the disease level, while the acetylcholinesterase (AChE), monoamine oxidase-A (MAO-A), and 5-hydroxytryptamine receptor 6 models were trained for specific AD targets. All four models performed excellently and were used to identify potential AD agents in the Kaixinsan formula. High-scoring compounds underwent experimental validation at the enzyme, cellular, and animal levels. Compounds like 2,4-di-tert-butylphenol and elemicin showed significant binding and inhibitory effects on AChE and MAO-A. Additionally, 13 compounds, including α-asarone, penetrated the blood-brain barrier, indicating potential brain target binding, and eight compounds enhanced microglial β-amyloid phagocytosis, aiding in clearing AD pathological substances. Our results demonstrate the effectiveness of deep learning models in developing AD therapies and provide a strong platform for AD drug discovery.