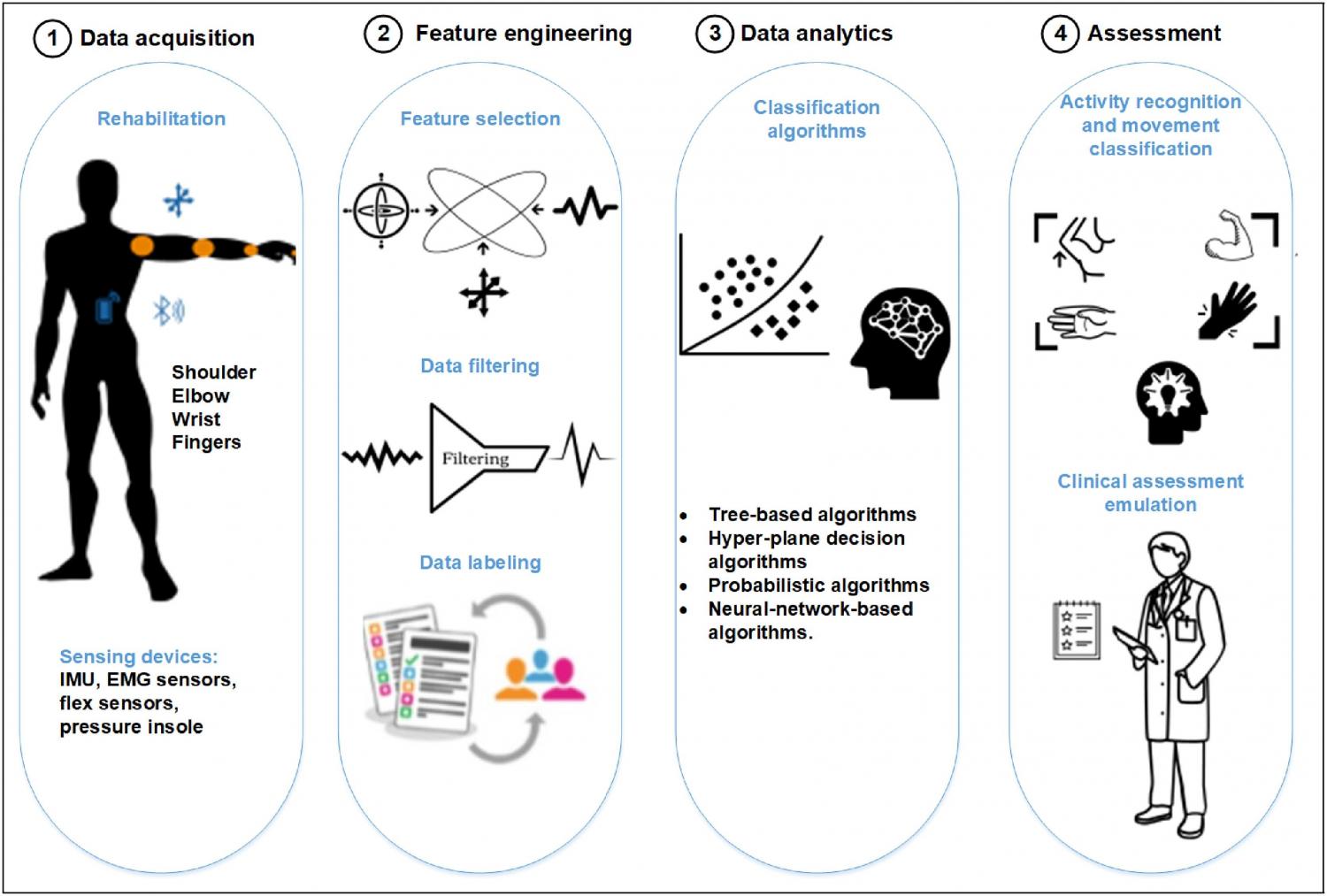
Elsevier, Biomedical Signal Processing and Control, Volume 71, January 2022
A cerebrovascular accident or stroke is the second commonest cause of death in the world. If it is not fatal, it can result in paralysis, sensory impairment and significant disability. Rehabilitation plays an important role to help survivors relearn lost skills and assist them to regain independence and thus ameliorate their quality of life. With the development of technology, researchers have come up with new solutions to assist clinicians in monitoring and assessing their patients; as well as making physiotherapy available to all. The objective of this review is to assess the recent developments made in the field of post-stroke rehabilitation using wearable devices for data collection and machine learning algorithms for the exercises’ evaluation. To do so, PRISMA guidelines for systematic reviews were followed. Scopus, Lens, PubMed, ScienceDirect and Microsoft academic were electronically searched. Peer-reviewed papers using sensors in post-stroke rehabilitation were included, for the period between 2015 to August 2021. Thirty-three publications that used wearable sensors for patients’ assessment were included. Based on that, we have proposed a taxonomy that divided the assessment systems into three categories namely activity recognition, movement classification, and clinical assessment emulation. Moreover, The most commonly employed sensors as well as the most targeted body–limbs, outcome measures, and study designs are reviewed, in addition to the examination of the machine learning approaches starting from the feature engineering to the classification done. Finally, limitations and potential study directions in the field are presented.